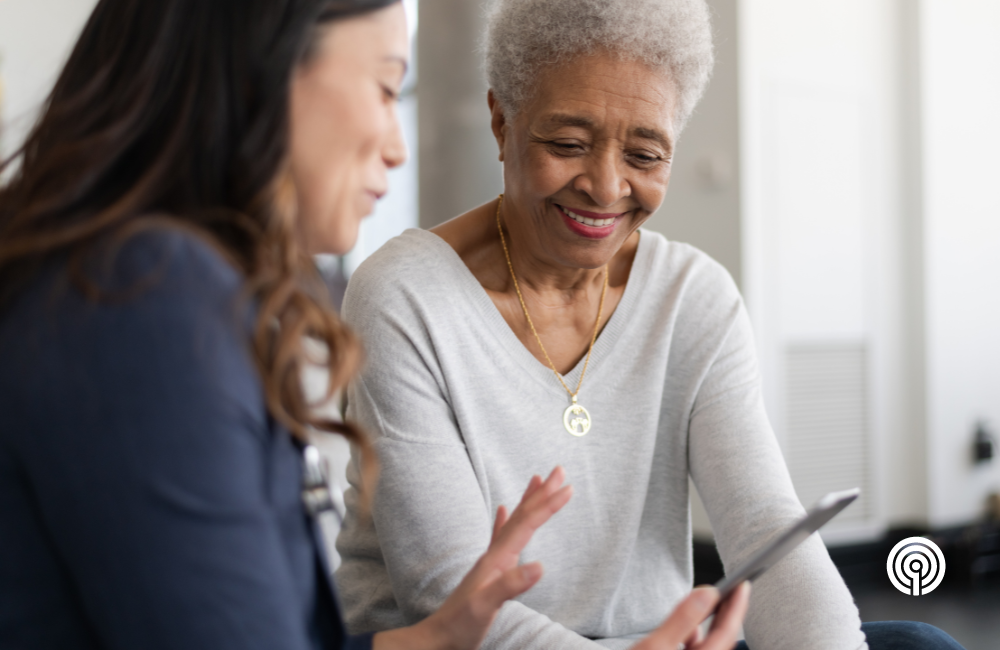
In today's evolving healthcare landscape, understanding and addressing structural bias is an ethical and practical necessity for healthcare organizations. But what exactly is structural bias in healthcare? Structural bias refers to the systemic and institutional practices and policies that create and perpetuate inequalities in healthcare access, treatment, and outcomes for different groups of people. For example, racial bias in healthcare algorithms can prioritize White patients over Black patients for certain treatments due to historical data imbalances.
The roots of structural bias in healthcare are deep and complex, stemming from historical, social, and economic inequities. Structural bias manifests in various ways, such as racial and ethnic disparities in health outcomes, gender bias in medical research and treatment, or socio-economic barriers to accessing care. These structural biases are not always overt or intentional, but their impact is profound and far-reaching.
The impact of structural bias in healthcare
Structural bias in healthcare leads to various issues, including unequal access to medical services, disparities in the quality of care received, and differences in health outcomes. For example, research has consistently shown that racial and ethnic minorities often receive lower quality care than their White counterparts, even when insurance status, income, age, and severity of conditions are comparable. Similarly, women's health problems have historically been under-researched, leading to gaps in knowledge and care.
Healthcare disparities affect the well-being of individuals and place an additional burden on the healthcare system as a whole. They contribute to higher costs due to delayed care, increased complications, and the need for more intensive treatments.
Addressing structural bias in healthcare
Healthcare organizations have a critical role in identifying, understanding, and mitigating the effects of structural bias. This begins with a commitment to equity and inclusion at all levels of the organization. But beyond commitment, there is a need for concrete actions. This is where online cultural competency and implicit bias training come into play.
Cultural competency and implicit bias training
Online training in cultural competency and implicit bias is an effective way to educate healthcare workers about the existence and effects of structural biases. Self-guided online courses can:
- Increase Awareness: They help identify personal biases and understand how these biases can influence decision-making and patient interactions.
- Promote Inclusive Practices: Training provides healthcare professionals with the tools and strategies to adopt more inclusive practices.
- Foster Empathy and Understanding: Courses help healthcare professionals understand and empathize with patients from diverse backgrounds, improving patient care and satisfaction.
Why online cultural competency training?
A self-guided online format offers several advantages for busy healthcare professionals:
- Flexibility: Healthcare workers can complete the training at their own pace and on their schedule.
- Scalability: Online training can be easily scaled to accommodate large numbers of employees across different locations.
- Consistency: Online courses ensure that all team members receive the same level of training and information.
By investing in online cultural competency and implicit bias training, healthcare organizations can take a substantial step toward dismantling the systemic barriers perpetuating healthcare disparities.
Understanding and addressing structural bias in healthcare is essential for promoting a more equitable and effective healthcare system. Implementing cultural competency and implicit bias training is one of the steps healthcare organizations can take to address these deep-rooted issues. While just a part of a broader strategy, online training contributes significantly to raising awareness among healthcare professionals about their implicit biases and the diverse perspectives of the populations they serve.
For more on bias, read our posts: